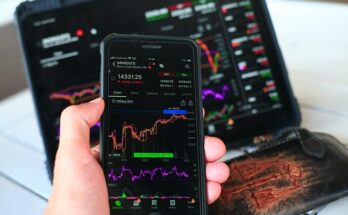
Revolutionizing Trading Strategies: Unraveling the Potential of SGML Trading Signals
January 14, 2023 Imphal By Banti Phurailatpam:
In the dynamic world of financial markets, staying ahead requires not only a keen understanding of market trends but also a mastery of innovative technologies. The latest buzz in the trading realm is the utilization of SGML (Standard Generalized Markup Language) in generating trading signals. This groundbreaking approach is reshaping how traders interpret market data, providing a new dimension to decision-making processes. In this article, we delve into the exciting world of SGML Trading Signals, exploring their potential, impact, and the future they hold for traders.
Understanding SGML in Trading
SGML, a versatile markup language designed for document structuring, may seem an unlikely candidate for revolutionizing trading strategies. However, its application in parsing and organizing complex financial data has garnered attention from tech-savvy traders and algorithmic trading enthusiasts. SGML’s ability to define document structure and relationships lays the foundation for creating advanced algorithms that can interpret market data with precision.
The Rise of Algorithmic Trading
Algorithmic trading has been a game-changer in the financial industry, enabling traders to execute orders at speeds and frequencies impossible for manual trading. SGML Trading Signals take algorithmic trading to the next level by leveraging SGML’s capabilities in parsing and structuring vast datasets. Algorithms equipped with SGML can process and interpret market information in a more granular and context-aware manner, potentially enhancing the accuracy of trading signals.
Parsing Financial Data with SGML
One of the key strengths of SGML lies in its ability to define and structure data elements within a document. In the context of financial markets, this means parsing and organizing complex datasets into meaningful components. SGML Trading Signals employ advanced parsing algorithms to break down financial information, such as market prices, indicators, and news, into structured elements. This structured data serves as the basis for generating more informed trading signals.
Context-Aware Decision Making
Traditional trading signals often rely on isolated indicators, sometimes overlooking the broader market context. SGML Trading Signals introduce a context-aware approach by considering the relationships and hierarchies defined in the parsed data. This nuanced understanding allows algorithms to factor in a more comprehensive view of the market, potentially leading to more informed and contextually relevant trading decisions.
SGML and Natural Language Processing
Incorporating Natural Language Processing (NLP) into trading algorithms is not a new concept, but SGML adds an extra layer of structure to this process. By using SGML to define the markup of financial news articles, reports, and social media sentiments, trading algorithms can extract and prioritize information more effectively. This integration of SGML and NLP enhances the depth and accuracy of sentiment analysis, a crucial factor in modern trading strategies.
Enhancing Signal Accuracy and Robustness
The ultimate goal of any trading signal is accuracy, and SGML Trading Signals aim to achieve just that. By structuring and organizing financial data with SGML, algorithms can identify subtle patterns, correlations, and anomalies that might elude traditional signal generation methods. The result is a potentially more robust and accurate set of signals, empowering traders to make well-informed decisions in fast-paced market conditions.
Challenges and Considerations
While the concept of SGML Trading Signals holds immense promise, it is not without challenges. Implementing SGML in trading algorithms requires a deep understanding of both financial markets and markup languages. Additionally, the dynamic nature of financial data poses challenges in maintaining the accuracy and relevance of structured information. Traders and developers venturing into SGML Trading Signals need to navigate these complexities to unlock the full potential of this innovative approach.
Future Implications and Trends
As SGML Trading Signals gain traction, their future implications on trading strategies and financial markets are intriguing. The convergence of structured data, algorithmic processing, and context-aware decision-making could pave the way for a new era in trading technology. The continuous evolution of SGML-based algorithms and their integration with emerging technologies may redefine how traders interact with and respond to market dynamics.
Conclusion: SGML’s Role in Shaping the Future of Trading
In the ever-evolving landscape of financial markets, innovation is the key to staying competitive. SGML Trading Signals represent a novel approach to interpreting and utilizing vast datasets in trading algorithms. As traders explore the potential of SGML, the synergy between structured markup languages and algorithmic trading may open new frontiers in signal accuracy, context-aware decision-making, and overall trading performance. The journey has just begun, and the future holds exciting possibilities for traders embracing SGML in their quest for financial success.
Revolutionizing Trading Strategies: Unraveling the Potential of SGML Trading Signals Read More